This chapter involves essentially one concept: establishing the significance of the simple total score for a person in the dichotomous RM. In both CTT and in Rasch measurement theory (RMT) , total scores play a special role. In CTT they do so by definition; in the dichotomous RM, they do as a consequence of the specification of the interaction between a person and an item. In this chapter there is an application of Eqs. (7.1) and (7.2) from the previous chapter, to show that the total score is a sufficient statistic .
The material in this chapter is not very easy. However, it is important, and it seems that there is no way to make it very easy. It is very simple at one level, and this simplicity also makes it sophisticated at another level. You will need to work through it a few times. The illustrations at the end of the chapter consolidate the concept of sufficiency.
The Total Score as a Sufficient Statistic

Possible response patterns and total scores for the responses of one person to two items i = 1 and i = 2
Item 1 |
Item 2 |
Total score |
---|---|---|
0 |
0 |
0 |
1 |
0 |
1 |
0 |
1 |
1 |
1 |
1 |
2 |
The key feature of
Table 8.1
is that it has listed the total score of a person to the two items. Rather than
as we did in
CTT , we denote this score for person n,
. In the case of two items, the
total score
of person n is given by
.
Notice that there are two patterns which
give the total score , and only one pattern which gives
each of the total scores
or
.
In the case that both responses are the same, that is, there is only one pattern which gives the total score , there is no basis for distinguishing between the difficulties of the items. The possibility for distinguishing between their difficulties arises only when the responses are different.
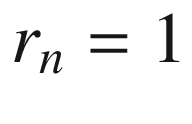

Thus is identical to
or
.

This notation, while convenient, is more
than convenient. Because the equation is independent of the person
parameter , it indicates that the total score of 1 contains all the information about the
person parameter and that there is no further information
about
in the
pattern.
Responses of a person to three items
Item 1 |
Item 2 |
Item 3 |
Total score |
---|---|---|---|
0 |
0 |
0 |
0 |
1 |
0 |
0 |
1 |
0 |
1 |
0 |
1 |
0 |
0 |
1 |
1 |
1 |
1 |
0 |
2 |
1 |
0 |
1 |
2 |
0 |
1 |
1 |
2 |
1 |
1 |
1 |
3 |
With 3 dichotomous items, the possible total scores are 0, 1, 2 and 3. Because there is only one pattern of responses that gives the extreme total scores, the scores of 0 and 3 (which are the minimum and maximum) provide no relative information about the items. However, given a score of either 1 or 2, there is more than one response pattern.
Following the argument for the case of two items, the following relationships can be established. We do not derive them here as the algebra is a little unwieldy, but it is shown in Andrich (1988) on pages 34–40.
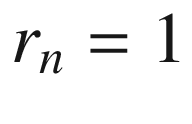
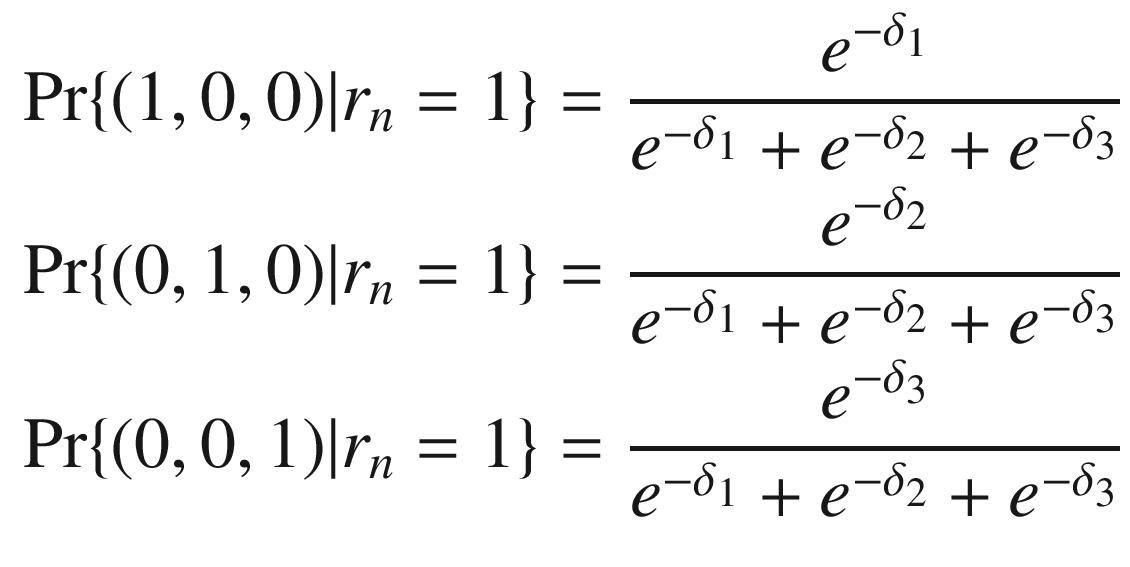
First, notice that the denominator in the three sub-equations of Eq. (8.3) is the same and is the sum of the numerators of these equations. This structure ensures that the sum of these conditional probabilities is 1, as they must be as the probability of all possible outcomes.
Second, again the sub-equations of
Eq. (8.3)
do not contain the proficiency of the person. That means, again,
that the total score of the person
contains all of the information about the person, and that the
response pattern does not contain any further information about the
person’s proficiency
. This is a property of the model, and
for it to hold in responses, the responses need to conform to the
dichotomous RM. How this conformity of responses to the model is
checked is a substantial part of later chapters of the book. The
check of this conformity between the responses and the model is
referred to as a test of fit .
By a symmetrical argument, it can be shown that the total score of an item is the key statistic containing all of the information about the difficulty of any item. You need to think about this a little. It is both a simple and very sophisticated concept; it took the genius of Sir Ronald Fisher, a statistician and geneticist, to formulate the concept of sufficiency. It is the cornerstone of Rasch models, and in his book Rasch (1960) says it was the high mark of Fisher’s contribution. Therefore, do not expect to understand sufficiency completely on your first reading.
Third, by containing all the information
of the proficiency of person n, the total
score is the basis for estimating
. Thus in the dichotomous RM, the
total score emerges as the key statistic with
information about the proficiency
. This is the same
as in CTT , where the total score
is simply assumed to contain
all of the information. However, because it emerges from a
different formulation, some other properties
different from CTT also emerge in the dichotomous RM. We
study these differences in the remainder of the book.
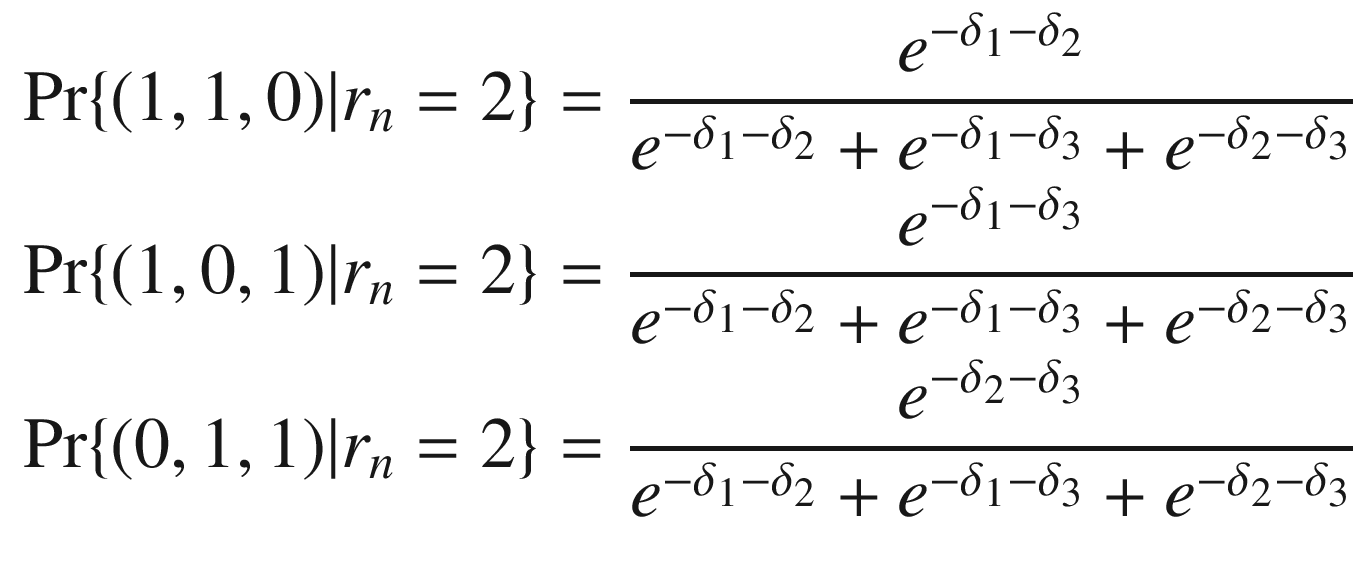
Notice again that the denominator of the sub-equations of Eq. (8.4) is the same and that it is the sum of the numerators of these equations. These equations become more complicated as the number of items increases. They are now handled in software either directly or indirectly.
The important point to note is that
these equations do not contain the person proficiency parameter
. We repeat the idea that given the
total score for a person, the probability
of the response does not depend on the person’s proficiency, but
only on the relative difficulties of the items. Therefore, all of
the information about the proficiency must be absorbed in the
total score , and there is no further
information about the person’s proficiency in the response
patterns.
Both results summarized in the above paragraph, (i) that the conditional probabilities given the total score do not involve person parameters, and (ii) that the total score contains all the information of a person’s proficiency, are used in analysing responses with the dichotomous RM.
The major consequence of the above derivations is that all persons with the same total score (irrespective of pattern of answers) will be given the same proficiency estimate. This is exactly as in CTT , but as noted earlier it is a consequence of the Rasch model and not by definition. One might ask the following question: given that the proficiencies of persons with the same total score are the same, what are the advantages of analysing the responses using the Rasch model? You will appreciate some of the advantages by the end of the first part of this book.
The Response Pattern and the Total Score
There is a common question asked by people when they first become acquainted with the Rasch model, although for some reason they do not ask this question in CTT , though it could be asked just as legitimately. The question: if all people with the same total score get the same proficiency estimate irrespective of the response pattern, is there not an injustice for persons who answer more difficult items correctly? Should not persons who answer more difficult items correctly have a greater proficiency estimate than those who answer the easy ones correctly? Before reading on, can you provide arguments against any injustice?
- (a)
The informal argument against any injustice
- (b)
The formal argument against any injustice
Example of conditional probabilities of 4
items, ,
,
,
1 |
Item 2 |
3 |
4 |
Total score |
Probability of pattern given total score |
---|---|---|---|---|---|
0 |
0 |
0 |
0 |
0 |
1.000a |
1 |
0 |
0 |
0 |
1 |
0.644a |
0 |
1 |
0 |
0 |
1 |
0.237 |
0 |
0 |
1 |
0 |
1 |
0.087 |
0 |
0 |
0 |
1 |
1 |
0.032 |
1.000 |
|||||
1 |
1 |
0 |
0 |
2 |
0.586a |
1 |
0 |
1 |
0 |
2 |
0.216 |
1 |
0 |
0 |
1 |
2 |
0.079 |
0 |
0 |
1 |
1 |
2 |
0.011 |
0 |
1 |
1 |
0 |
2 |
0.079 |
0 |
1 |
0 |
1 |
2 |
0.029 |
1.000 |
|||||
1 |
1 |
1 |
0 |
3 |
0.644a |
1 |
1 |
0 |
1 |
3 |
0.237 |
1 |
0 |
1 |
1 |
3 |
0.087 |
0 |
1 |
1 |
1 |
3 |
0.032 |
1.000 |
|||||
1 |
1 |
1 |
1 |
4 |
1.000 a |
It can be seen in Table 8.3 that given each total score , each response pattern has a probability of occurring, and that with items with different difficulty, these probabilities are different. In the table, these probabilities are ordered for each total score , with the highest probability first. The pattern with the highest probability for each total score should be familiar. Can you see what it is before reading on?
Patterns from Table 8.3 with the greatest conditional probabilities
1 |
Item 2 |
3 |
4 |
Total Score |
Probability of pattern given total score |
---|---|---|---|---|---|
0 |
0 |
0 |
0 |
0 |
1.000a |
1 |
0 |
0 |
0 |
1 |
0.694a |
1 |
1 |
0 |
0 |
2 |
0.586a |
1 |
1 |
1 |
0 |
3 |
0.644a |
1 |
1 |
1 |
1 |
4 |
1.000 a |
The results in Table 8.4 show that even if responses fit the Rasch model, we will not always get a Guttman pattern. If they fit, then we are most likely to get Guttman patterns, but we will get the other patterns as well, with probabilities that can be calculated. Thus in the example of Table 8.3, even if the responses fitted the Rasch model, we would expect that of the people who had a total score of 2, some 21.6% would have the response pattern (1, 0, 1, 0). However, if a lot more people with a total score of 2 had this response pattern, we would have to say that the responses do not fit the Rasch model. We would have to conclude that the total score is not a sufficient statistic for the proficiency, and that the total score cannot be used to infer a single proficiency for the person. There indeed is information in the pattern of responses.
The point, then, is that the response patterns, in the case that they fit the dichotomous Rasch model, are very likely to be close to the Guttman pattern (though not perfectly) and in the case of patterns close to the Guttman pattern, there is no further information in the profile other than that in the total score . Diagnosing where the response patterns do not fit the Rasch model is central to the analysis of responses according to the dichotomous RM. We study some aspects of this diagnosis in the chapters on fit of data to the model .