Statistics Review 9: Independence
In this chapter, we emphasize that independence of responses, formalized in the Rasch model, is a requirement for fit to the model. We outline the different ways independence can be violated, how these violations have been formalized, methods of detection, and describe the effects of violations of this assumption on estimates. In Chap. 24: Violations of the Assumption of Independence II—The Polytomous Rasch Model more methods of detection are outlined, most of which use the polytomous Rasch model (PRM).
Local Independence
The statistical independence formalized in the Rasch model reflects the intentions of test developers when constructing and assembling items. The same independence is implied in CTT . The Rasch model is typically used for analyses of the psychometric properties of scales or tests in which responses to a number of different items are summed. They are summed because they are considered to capture a unidimensional construct. The summed responses of more than one item should be more valid and reliable than a response to one item only. However, this is true only when each item measures the same trait as the other items in the scale and provides some unique information not provided by the other items. In other words, in order to provide a reliable scale of summed items each item needs to provide related but independent information, or relevant but not redundant information. An analysis according to the Rasch model will reveal, as an anomaly , items that do not provide relevant or independent information.
![$$ { \Pr }\{ X_{ni} = x\} = [{ \exp }(x(\beta_{n} - \delta_{i} ))]/[1 + { \exp }(\beta_{n} - \delta_{i} )] $$](/epubstore/A/D-Andrich/A-Course-In-Rasch-Measurement-Theory/OEBPS/images/470896_1_En_14_Chapter/470896_1_En_14_Chapter_TeX_Equ1.png)








The holding of Eqs. (14.1) and (14.2) together is
generally referred to as local independence (Lazarsfeld
& Henry, 1966; Andrich, 1991).
The term local refers to the
idea that all the variation among responses to an item is accounted
for by the person parameter , and therefore that for the same
value of
, there is no further relationship
among responses.
In the Rasch model, the person parameter
is the source of general dependence among responses to
items in the sense that a person with a high value of
will tend to respond positively to
all items, and the opposite for a person with a low value of
. In the estimation of the item
parameters,
can be eliminated. With this
parameter eliminated, or for the same value of
, there should be no further
relationship among the items. The absence of this kind of
relationship is referred to as local independence .
Two Violations of Local Independence
Local
independence in Rasch models defined as above can be
violated in two generic ways. First, there may be person parameters
other than that are involved in the response.
This is a violation of unidimensionality and therefore statistical independence relative to the model of
Eq. (14.1).
Second, for the same person and
therefore the same value of , the response to one item might
depend on the response to a previous item. This is a violation of
statistical independence relative to
Eq. (14.2). To distinguish this latter violation of
Eq. (14.2) from the violation of unidimensionality,
we refer to the latter as response dependence . Both these
violations have been formalized algebraically in Marais and Andrich
(2008a, b). The papers also provide some
examples of these two types of violations of independence in
practice.
Multidimensionality
Many scales in psychology, education and social measurement in general, which are constructed to measure a single variable, are nevertheless composed of subsets of items which measure different but related aspects of the variable. An example is the Functional Independence Measure (FIM™) motor scale (Keith, Granger, Hamilton, & Sherwin, 1987), which consists of 13 items, ranging from bladder management to climbing stairs. These items can be grouped into subsets, for example, Sphincter Control can comprise Bowel Management and Bladder Management. Although the presence of subsets captures better the complexity of a variable and increases its validity , it compromises the model’s unidimensionality. Another example is the Australian Scholastic Aptitude Test (ASAT) where items, which are summed, are grouped into subsets representing mathematics, science, humanities and social science (Bell, Pattison, & Withers, 1988).
Multidimensionality is also found in items that are linked by attributes such as common stimulus materials, common item stems, common item structures or common item content. These have been described as subtests (Andrich, 1985), testlets (Wang, Bradlow, & Wainer, 2002) or item bundles (Rosenbaum, 1988; Wilson & Adams, 1995).
Formalization of Multidimensionality

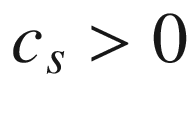


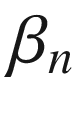
Therefore, is the value of the main, common
variable or trait among subsets, and
is the variable or trait unique to
each subset. The value
characterizes the magnitude of the
variable of subset s relative
to the common variable among subsets.





Unidimensional

Multidimensional
Summary of subset design
Items |
Subsets |
|||
---|---|---|---|---|
1 |
2 |
… |
S |
|
1 |
|
|
… |
|
2 |
|
|
… |
|
⋮ |
⋮ |
⋮ |
⋮ |
⋮ |
I |
|
|
… |
|
Detection of Multidimensionality
Individual Item Fit
Violations of independence will be reflected in the fit of data to the model . In general, over-discriminating items often indicate response dependence and under-discriminating items often indicate multidimensionality . Response dependence increases the similarity of the responses of persons across items and responses are then more Guttman-like than they should be under no response dependence . Multidimensionality acts as an extra source of variation (noise) in the data and the responses are less Guttman-like than they would be under no dependence.
Correlations of Standardized Residuals Between Items
Violations of local independence can be further assessed by examining patterns among the standardized item residuals. High correlations between standardized item residuals indicate a violation of local independence .
Principal Component Analysis (PCA ) of the Item Residuals
A principal component analysis (PCA ) of the item residuals provides further information about multidimensionality . After accounting for the single dimension of the items by the Rasch model, there should be no further pattern among the residuals. If a PCA indicates a meaningful pattern for the scale or test , it can indicate a lack of unidimensionality. It can also indicate response dependence considered in the next section. The context needs to be used to decide the source of the correlation.
Results of a PCA , items sorted according to their loadings on PC1
RUMM2030 Project: MD2 Analysis: RUN1 |
||||||||||
Title: RUN1 |
||||||||||
Display: PC loadings |
||||||||||
Item |
PC1 |
PC2 |
PC3 |
PC4 |
PC5 |
PC6 |
PC7 |
PC8 |
PC9 |
PC10 |
I0008 |
0.392 |
−0.098 |
−0.271 |
−0.200 |
−0.148 |
0.112 |
−0.005 |
−0.325 |
0.106 |
0.083 |
I0010 |
0.370 |
−0.083 |
0.120 |
−0.030 |
0.049 |
−0.375 |
−0.004 |
−0.200 |
0.165 |
0.080 |
I0009 |
0.369 |
0.007 |
−0.170 |
−0.097 |
0.188 |
−0.061 |
0.313 |
−0.202 |
−0.176 |
−0.395 |
I0006 |
0.363 |
−0.167 |
0.346 |
−0.107 |
−0.072 |
−0.267 |
−0.127 |
0.115 |
0.041 |
0.049 |
I0005 |
0.346 |
−0.260 |
−0.030 |
−0.384 |
−0.039 |
0.116 |
−0.183 |
−0.181 |
−0.200 |
0.139 |
I0001 |
0.325 |
0.042 |
0.230 |
−0.158 |
0.153 |
−0.084 |
0.082 |
−0.033 |
−0.197 |
−0.146 |
I0007 |
0.320 |
0.299 |
−0.106 |
0.017 |
0.162 |
−0.246 |
0.063 |
0.217 |
0.188 |
0.254 |
I0011 |
0.303 |
−0.119 |
−0.039 |
0.304 |
−0.216 |
−0.065 |
−0.080 |
−0.194 |
0.019 |
−0.308 |
I0015 |
0.289 |
−0.015 |
0.015 |
0.401 |
−0.345 |
−0.038 |
0.077 |
0.041 |
0.034 |
0.319 |
I0013 |
0.282 |
−0.059 |
−0.150 |
−0.017 |
0.073 |
0.386 |
−0.308 |
0.278 |
−0.292 |
−0.114 |
I0012 |
0.262 |
0.057 |
−0.380 |
0.180 |
0.197 |
−0.070 |
0.187 |
0.090 |
−0.024 |
0.220 |
I0002 |
0.256 |
−0.187 |
0.131 |
0.166 |
−0.301 |
0.306 |
0.173 |
0.311 |
−0.208 |
−0.096 |
I0003 |
0.251 |
0.458 |
−0.040 |
−0.137 |
−0.141 |
0.198 |
0.091 |
0.107 |
0.089 |
0.031 |
I0014 |
0.250 |
0.066 |
−0.180 |
0.015 |
0.225 |
0.022 |
−0.373 |
0.244 |
0.428 |
−0.116 |
I0004 |
0.195 |
0.127 |
0.621 |
0.219 |
0.164 |
0.219 |
−0.003 |
−0.003 |
0.042 |
0.034 |
I0030 |
−0.193 |
0.532 |
−0.045 |
−0.095 |
−0.198 |
0.032 |
−0.182 |
−0.065 |
−0.185 |
−0.114 |
I0016 |
−0.231 |
−0.030 |
−0.133 |
0.287 |
0.100 |
−0.356 |
0.036 |
−0.084 |
−0.385 |
0.119 |
I0028 |
−0.236 |
0.481 |
0.208 |
−0.116 |
−0.108 |
0.003 |
0.113 |
0.040 |
0.151 |
−0.074 |
I0029 |
−0.266 |
0.057 |
−0.071 |
−0.141 |
0.025 |
−0.311 |
−0.093 |
0.466 |
−0.354 |
−0.110 |
I0019 |
−0.271 |
−0.087 |
−0.248 |
0.425 |
0.106 |
0.189 |
0.001 |
−0.062 |
0.224 |
−0.114 |
I0025 |
−0.302 |
0.080 |
0.051 |
−0.122 |
0.210 |
0.225 |
0.026 |
−0.137 |
−0.198 |
0.487 |
I0027 |
−0.306 |
−0.323 |
0.167 |
−0.062 |
−0.164 |
−0.068 |
−0.047 |
0.281 |
0.269 |
0.013 |
I0017 |
−0.307 |
0.042 |
−0.182 |
−0.350 |
−0.024 |
−0.032 |
0.157 |
−0.044 |
0.253 |
−0.168 |
I0026 |
−0.309 |
0.146 |
0.290 |
0.198 |
0.233 |
0.042 |
−0.259 |
−0.370 |
−0.038 |
−0.062 |
I0018 |
−0.338 |
0.131 |
−0.224 |
0.195 |
−0.190 |
−0.094 |
−0.353 |
−0.046 |
−0.084 |
0.077 |
I0021 |
−0.343 |
−0.238 |
0.092 |
−0.019 |
0.436 |
−0.109 |
0.044 |
0.136 |
0.017 |
−0.083 |
I0022 |
−0.347 |
0.006 |
0.077 |
0.024 |
−0.360 |
−0.158 |
0.374 |
−0.023 |
−0.029 |
−0.103 |
I0024 |
−0.361 |
−0.226 |
−0.015 |
−0.241 |
−0.141 |
0.095 |
0.026 |
−0.021 |
0.062 |
0.345 |
I0023 |
−0.362 |
−0.159 |
0.008 |
−0.097 |
−0.211 |
−0.059 |
−0.316 |
−0.132 |
0.034 |
−0.172 |
I0020 |
−0.390 |
−0.133 |
−0.055 |
0.052 |
0.178 |
0.315 |
0.319 |
0.038 |
0.057 |
−0.080 |
Summary of the PCA in Table 14.2
RUMM2030 Project: MD2 Analysis: RUN1 |
||||
Title: RUN1 |
||||
Display: Principal component summary |
||||
PC |
Eigen |
Percent (%) |
CPercent (%) |
StdErr |
PC001 |
2.869 |
9.56 |
9.56 |
0.402 |
PC002 |
1.317 |
4.39 |
13.95 |
0.175 |
PC003 |
1.251 |
4.17 |
18.12 |
0.165 |
PC004 |
1.209 |
4.03 |
22.15 |
0.164 |
PC005 |
1.163 |
3.88 |
26.03 |
0.159 |
PC006 |
1.126 |
3.75 |
29.78 |
0.153 |
PC007 |
1.097 |
3.66 |
33.44 |
0.149 |
PC008 |
1.084 |
3.61 |
37.05 |
0.146 |
PC009 |
1.083 |
3.61 |
40.66 |
0.147 |
PC010 |
1.065 |
3.55 |
44.21 |
0.143 |
PC011 |
1.047 |
3.49 |
47.70 |
0.138 |
PC012 |
1.009 |
3.36 |
51.06 |
0.136 |
PC013 |
1.003 |
3.34 |
54.41 |
0.135 |
PC014 |
0.974 |
3.25 |
57.66 |
0.128 |
PC015 |
0.959 |
3.20 |
60.85 |
0.129 |
PC016 |
0.933 |
3.11 |
63.96 |
0.127 |
PC017 |
0.929 |
3.10 |
67.06 |
0.127 |
PC018 |
0.911 |
3.04 |
70.09 |
0.124 |
PC019 |
0.902 |
3.01 |
73.10 |
0.122 |
PC020 |
0.885 |
2.95 |
76.05 |
0.122 |
PC021 |
0.860 |
2.87 |
78.91 |
0.117 |
PC022 |
0.837 |
2.79 |
81.70 |
0.113 |
PC023 |
0.830 |
2.77 |
84.47 |
0.114 |
PC024 |
0.811 |
2.70 |
87.17 |
0.110 |
PC025 |
0.801 |
2.67 |
89.84 |
0.109 |
PC026 |
0.772 |
2.57 |
92.41 |
0.106 |
PC027 |
0.740 |
2.47 |
94.88 |
0.102 |
PC028 |
0.725 |
2.42 |
97.30 |
0.098 |
PC029 |
0.706 |
2.35 |
99.65 |
0.096 |
PC030 |
0.104 |
0.35 |
100.00 |
0.030 |
Other Tests of Multidimensionality
If a PCA indicates that the residuals pattern into more than one subscale, RUMM2030 provides additional tests of unidimensionality. In Chap. 24: Violations of the Assumption of Independence II—The Polytomous Rasch Model a method for estimating the magnitude of multidimensionality , c in Eq. (24.2), is discussed. This method makes use of the polytomous Rasch model . Another method for testing the equivalence of two subsets of items, hypothesized to measure two different dimensions, is introduced.
Response Dependence
A second violation of the assumption of independence is response dependence . Response dependence occurs when a person’s response to an item depends on the person’s response to a previous item. This can occur in cases where a correct answer on a question gives a clue or the answer to one or more subsequent questions. Or the case where the structure of different questions is such that an answer to one question logically implies the answer to another question. Kreiner and Christensen (2007) show that the items Climbing one flight of stairs and Climbing several flights of stairs of the physical functioning subscale of the SF-36, a widely used rating scale in health research, are response dependent. Similarly, the items Walking one block, Walking several blocks and Walking more than a mile are dependent in this way. They should be different levels of one ordered category item. Another example is where judges make decisions on multiple criteria with respect to some object and a halo effect operates across all criteria.
Formalization of Response Dependence
Equation (14.5) does not satisfy
Eq. (14.4). The value d characterizes the magnitude of response
dependence . A correct response = 1 by person n to item i reduces the difficulty of item
j to
thus increasing the probability of
the same correct response
= 1 to item j. Similarly, the response
= 0 on item i increases the difficulty of item
j to
, thus increasing the probability of
the same incorrect response
= 0 to item j.
Detection of Response Dependence
Individual Item Fit
Violations of independence will be reflected in the fit of data to the model . In general, over-discriminating items often indicate response dependence and under-discriminating items often indicate multidimensionality . Response dependence increases the similarity of the responses of persons across items and responses are then more Guttman-like than they should be under no dependence. Multidimensionality acts as an extra source of variation (noise) in the data, and the responses are less Guttman-like than they would be under no dependence.
Correlations Between Standardized Item Residuals
Correlations between standardized item residuals (only the first ten items are shown due to space restrictions)
RUMM2030 Project: RD Analysis: R |
||||||||||
Title: R |
||||||||||
Display: Residual correlation matrix |
||||||||||
Item |
I0001 |
I0002 |
I0003 |
I0004 |
I0005 |
I0006 |
I0007 |
I0008 |
I0009 |
I0010 |
I0001 |
1.000 |
|||||||||
I0002 |
−0.057 |
1.000 |
||||||||
I0003 |
−0.026 |
−0.061 |
1.000 |
|||||||
I0004 |
−0.012 |
−0.008 |
−0.057 |
1.000 |
||||||
I0005 |
−0.038 |
−0.044 |
−0.080 |
0.481 |
1.000 |
|||||
I0006 |
−0.053 |
−0.011 |
−0.017 |
−0.112 |
−0.127 |
1.000 |
||||
I0007 |
−0.078 |
−0.065 |
−0.006 |
−0.019 |
−0.005 |
−0.038 |
1.000 |
|||
I0008 |
−0.080 |
0.017 |
−0.051 |
−0.047 |
−0.058 |
−0.002 |
−0.054 |
1.000 |
||
I0009 |
−0.022 |
−0.008 |
−0.053 |
−0.081 |
−0.075 |
−0.033 |
0.015 |
−0.030 |
1.000 |
|
I0010 |
0.071 |
0.006 |
−0.036 |
−0.099 |
−0.065 |
−0.042 |
−0.039 |
−0.035 |
−0.029 |
1.000 |
Estimating the Magnitude of Response Dependence
Andrich and Kreiner (2010) describe a way of estimating the value of d in Eq. (14.5), which characterizes the magnitude of response dependence . It is estimated as a change in the location of the difficulty of item j caused by its dependence on item i. The focus is on dependence between two dichotomous items.
Individual item fit statistics for items 5S0 and 5S1 in the case of dependence
Seq |
Item |
Type |
Location |
SE |
FitResid |
DF |
ChiSq |
DF |
Prob |
---|---|---|---|---|---|---|---|---|---|
31 |
005S0 |
Poly |
−0.618 |
0.196 |
−0.973 |
171.68 |
11.779 |
9 |
0.226 |
32 |
005S1 |
Poly |
−4.865 |
0.296 |
−0.294 |
763.87 |
6.202 |
9 |
0.720 |

The estimated magnitude of = (−0.618 – (−4.865))/2 = 2.12 is
very close to the value of
used to simulate the data set.
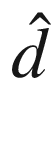
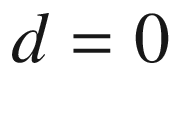
Individual item fit statistics for items 5S0 and 5S1 in the case of no dependence
Seq |
Item |
Type |
Location |
SE |
FitResid |
DF |
ChiSq |
DF |
Prob |
---|---|---|---|---|---|---|---|---|---|
31 |
005S0 |
Poly |
−2.507 |
0.181 |
−1.270 |
172.64 |
7.171 |
9 |
0.619 |
32 |
005S1 |
Poly |
−2.355 |
0.123 |
−1.088 |
763.87 |
8.270 |
9 |
0.507 |
The Effects of Violations of Independence

Person and item distributions for both types of dependence as well as no dependence