Motivations and Background to CTT and RMT
In this chapter, we summarize some comparisons and contrasts between Classical Test Theory (CTT) and Rasch Measurement Theory (RMT) . Because the motivation of the theories and models appear so different we could take the position that the two theories are incompatible. However, although there are critical differences between the two, because of the way the theories are reflected in their assumptions and in their respective mathematical expressions as models, we take the position that RMT can be seen as an elaboration of CTT . We justify such a position in this chapter.
We begin by summarizing the very different motivations of the respective theories. We suggest that just because they have different motivations RMT can end up being an elaboration of CTT . We further suggest in a later chapter, which deals with general Item Response Theory (IRT) , that if one sets out to elaborate CTT directly, it does not lead to the kind of elaboration of CTT that RMT provides. In particular, it does not lead to the total score of a person on a set of items being the key statistic. Instead, the elaborations can be seen as an ad hoc addition of parameters to account better for different data sets.
Motivation of CTT
CTT , which appeared early in the twentieth century, seems to have arisen from the following ingredients. First, from a substantive point of view, the emergence and formalization of testing, in particular, intelligence testing for assessing whether or not young children could profit from a regular education in this period. Second, the development and application of the correlation coefficient in the human sciences using simply summed scores on dichotomously scored items that provided a test score. Third, from the developments in the analysis of data, the acceptance that observations could show random variation, where random variation may be seen as an error. Further, theoretical developments of error variance lead to the normal distribution and its application with true and error scores being additive. Fourth, the idea that different dichotomously scored items of a test administered to a person could be seen as replications of each other in some sense. As a result of these being replications, the possibility of summing the random variables to give a total score for a person seemed to have been justified rather than simply assumed. However, and asymmetrically, it was understood that different persons have different proficiencies.
Motivation of RMT
As we have indicated already, the motivation for RMT is that within a frame of reference , the comparisons of persons and the comparisons of items are invariant with respect to different subsets of items and persons, respectively. The comparisons are in terms of characterizations of persons and items with real numbers. The history of Rasch’s development of his theory of measurement can be obtained from the foreword to his book, Probabilistic Models for Some Intelligence and Attainment Tests (1960), as well as from Andersen and Olsen (2001) and Andrich (2005).
As a consultant to the Danish Institute of Educational Research, Rasch was asked to help devise a study which would ascertain the effectiveness of a reading program for children who had reading difficulties. Instead of any conception of CTT , he approached the problem as he had done with research studies he worked on in biomedical and other research areas. There were different pieces of data collected, but the data set we refer to is the one in which the children read different texts out loud and the responses recorded were the errors they made in reading the words.
If the growth of students was to be assessed, then they had to be given texts to read that were not so difficult that the students would not engage with the reading, and not so easy that they would not make any errors at all. However, if they improved in their reading over time, then as their reading improved, they needed to be given more difficult texts. Therefore, the different texts of different difficulty had to be placed on the same scale .
Clearly, different words were of somewhat different difficulty within a text, but nevertheless, the texts were relatively homogeneous and were chosen to be of different overall difficulty. To place these texts on the same scale a linking design of the kind we saw in the last chapter was used. Thus, adjacent grades read common texts, and also texts that were of a relevant difficulty for the grade. Rasch characterized a response with a parameter for a person’s reading proficiency, and a parameter for a text’s reading difficulty. Because the error count was relatively small, he knew that the Poisson distribution had the potential to be useful in characterizing the distribution of responses. However, his use of the Poisson was distinctive—it was to characterize the error count of a particular person to a particular text, rather than a population of persons to a group of texts. Thus, he focused on the individual, and did not assume a normal distribution of persons as was done in CTT .
The references above describe how Rasch came to appreciate that his characterization provided the possibility of eliminating the person parameters while estimating the difficulties of the texts, and vice versa, and the formalization of the models that provided invariant comparisons . Rasch then worked out the model for dichotomous responses by extrapolating the response structure that would be required if each word was characterized for its difficulty, for each word to be read that would lead to the Poisson model for the text as a whole. He then applied it to two data sets he had at hand, a Danish intelligence test , and the nonverbal Ravens Progressive Matrices test . In the former, the responses did not conform to the model, in the latter they essentially did. In the former, he was able, from the study of fit, to diagnose different dimensions that were being assessed.
This is a brief summary of the way Rasch came upon the model for dichotomous responses, a way that was very different from the way CTT was developed. It was a model for dichotomous responses which had the property of sufficiency and the possibility of eliminating one set of parameters while estimating the others. This is the case for the model. It was not that it accounted for any data set. It came before any data were collected with its use in mind. However, one feature was common with CTT , that the items of a test assessed the same variable and that somehow, each person should be characterized by a single number.
Relating Characteristics of CTT and RMT
Some comparisons between CTT and RMT for dichotomous responses
CTT |
RMT |
|
---|---|---|
Motivation |
Multiple items are a form of replication in the assessment of a person |
Requirement: invariance of comparisons of items and persons relative to each other within a frame of reference |
Assumptions, requirements, and implications |
Unidimensionality, independent responses and a normal distribution of the variable in the population |
Unidimensionality, independent responses, but no assumption of the distribution of persons |
Item locations |
Outside the theory, no formalization In practice, a facility index referenced to a population |
Formalized as a difficulty Central to defining a continuum |
Algebraic formalization |
Equal correlation among all pairs of items in the population |
Equal slopes for the ICCs |
Key property |
Total score on a set of items is defined (asserted) to characterize a person |
The total score as
the sufficient statistic for |
Person estimation |
Linear relation between total score |
Non-linear relation between total score |
SE of the person estimate |
Same for all persons |
Variable depending on person and item locations and greater at extreme scores |
Reliability |
Ratio of the estimated true variance relative
to observed variance, e.g. coefficient |
Ratio of the estimated location variance relative to observed variance, e.g. the index of person separation calculated from variances of estimates and their standard errors |
Missing responses and linking /equating |
Missing responses generally imputed,
coefficient Equipercentile equating |
Missing responses are handled routinely, different persons can respond to different subsets of items and obtain proficiency estimates on the same scale |
Item discrimination |
Assumes common discrimination Individual item discrimination outside the theory In practice item discrimination is used as a fit index and low discrimination is a concern The greater the discrimination the better, though it is understood it can be too high, resulting from strong local dependence, which leads to a loss of validity It is known as the attenuation paradox |
Assumes common discrimination Individual item discrimination outside the theory In practice different discriminations observed from a fit to the model perspective The discrimination of items in each analysis sets the scale for the ICCs Items discriminating significantly greater than the average and relatively worse than the average are both of concern |
The Total Scores of Persons
We have seen that in CTT for dichotomous responses, each response to an item is scored 0 and 1 and that the sum of these scores is assumed to characterize a person. In RMT , the items are scored in the same way, and it turns out that as a consequence of the model, the total score of a person is the sufficient statistic for the person’s parameter estimate, and likewise for items.

Distribution of total scores
CTT Estimation of the True Score

Clearly, the estimate is referenced to
the mean of the group and to the reliability
of the instrument in that group. Furthermore, the
relationship between the true score
estimates and the raw scores is linear.
![$$ V[\hat{t}_{n} ] = r_{yy}^{2} V[y] $$](/epubstore/A/D-Andrich/A-Course-In-Rasch-Measurement-Theory/OEBPS/images/470896_1_En_12_Chapter/470896_1_En_12_Chapter_TeX_Equ2.png)
![$$ SD[\hat{t}_{n} ] = r_{yy}^{{}} SD[y] $$](/epubstore/A/D-Andrich/A-Course-In-Rasch-Measurement-Theory/OEBPS/images/470896_1_En_12_Chapter/470896_1_En_12_Chapter_TeX_IEq25.png)
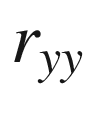
![$$ SD[\hat{t}_{n} ] = 0.604\left( {2.508} \right) = 1.514 $$](/epubstore/A/D-Andrich/A-Course-In-Rasch-Measurement-Theory/OEBPS/images/470896_1_En_12_Chapter/470896_1_En_12_Chapter_TeX_IEq27.png)
![$$ \begin{aligned} \hat{t}_{x + 1} - \hat{t}_{x} & = \bar{y} + r_{yy} (y_{x + 1} - \bar{y}) - [\bar{y} + r_{yy} (y_{x} - \bar{y})] \\ & = \bar{y} + r_{yy} y_{x + 1} - r_{yy} \bar{y} - \bar{y} - r_{yy} y_{x} + r_{yy} \bar{y} \\ & = r_{yy} y_{x + 1} - r_{yy} y_{x} \\ & = r_{yy} (y_{x + 1} - y_{x} ) \\ & = r_{yy} (1) \\ & = r_{yy} \\ \end{aligned} $$](/epubstore/A/D-Andrich/A-Course-In-Rasch-Measurement-Theory/OEBPS/images/470896_1_En_12_Chapter/470896_1_En_12_Chapter_TeX_Equ3.png)
That is, the difference between two successive raw scores has been shrunk by exactly the reliability .


Distribution of estimated true scores
Finally, because there is no parameter for an item to take account of its difficulty, for the above comparisons to be made, it is necessary that all persons have responded to the same items.
RMT Estimation of the Person Location Estimates

Non linear transformation of the total score to a Rasch model estimate
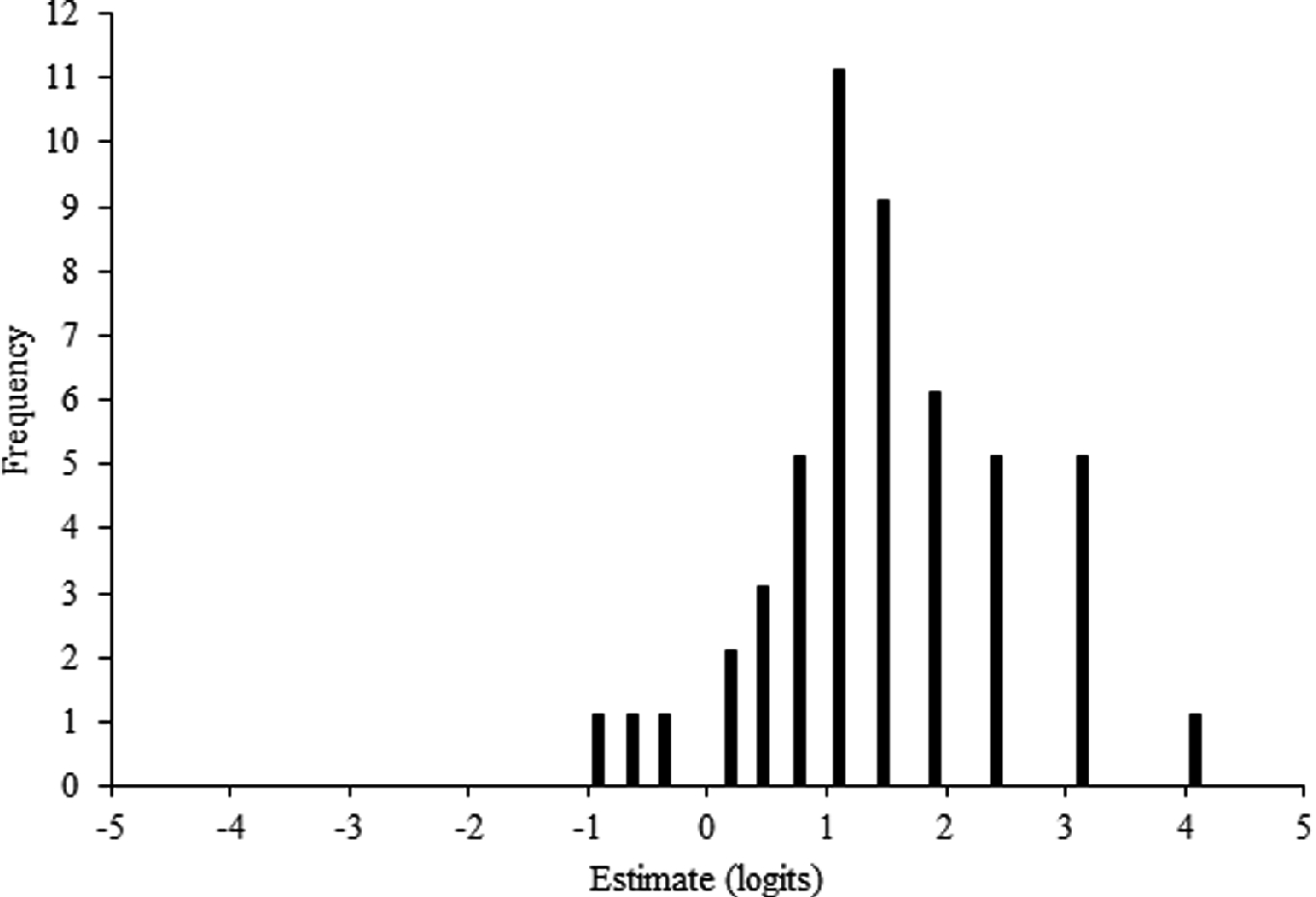
Distribution of the dichotomous Rasch model estimates
CTT Estimation of Standard Errors of True Scores


RMT Estimation of Standard Errors of Person Location Estimates
